Application of deep learning for detection and segmentation of tumor region of breast ultrasound images.
Adebayo Abdulganiyu KEJI || Olumide SANGOTOKI
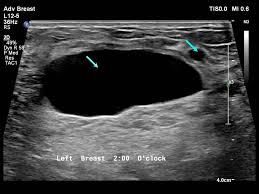
Abstract
Breast tumor has been a major medical condition or suffering from disease with high mortality rate popularly among women around the world. Normally, cells divide in an orderly and controlled way. However, If the procedure somehow goes awry, the cells will continue to divide and create a tumor. The cells lining the breast ducts tend to overgrow, which is the usual cause of breast tumors. The detection of this disease had gone through numerous numbers of research from traditional means to modern methods. However, dealing with life of an individual is so important that one need not to give room or appreciate lapses as a result of the method use in diagnosis and detection of high mortality related diseases. In this study, we proposed the utilization of an expanded-Unet deep neural network to segment breast tumors while preserving the edge features and texture data of the images of breast tumors. To increase the generalizability of the current breast tumor segmentation images, we used more training and validation dataset. Since the misrepresentation of breast tumors in the mask image used to train the segmentation model could have an impact on the training process' results, these images were carefully selected and cleaned. However, we achived a dice similarity score of 94.0, and an IOU of 99.0%. For our strategy, it is evident from the results that our suggested model performed better in terms of intersection over union and similarity of dice scores.
Methodology
In addition to the dataset, the experiment's procedure, and the assessment criteria, this introduces the technique that was used to conduct the study.
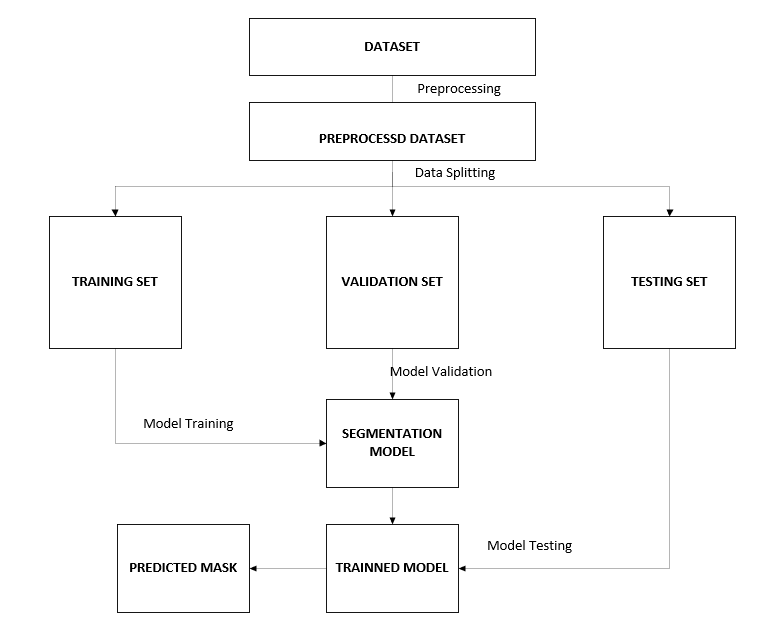
Conclusion
Breast tumor has been a major medical condition or suffering from disease with high mortality rate popularly among women around the world. Normally, cells divide in an orderly and controlled way. However, If the procedure somehow goes awry, the cells will continue to divide and create a tumor. The cells lining the breast ducts tend to overgrow, which is the usual cause of breast tumors. The detection of this disease had gone through numerous numbers of research from traditional means to modern methods. However, dealing with life of an individual is so important that one need not to give room or appreciate lapses as a result of the method use in diagnosis and detection of high mortality related diseases. In this study, we proposed the segmentation of breast tumor using Expanded-Unet deep neural network in order to preserve the edge characteristics and texture information of breast tumors images. We employed the use of more training and validation dataset to improve generalization of the existing breast tumor segmentation images. These images were carefully selected/cleaned in order to solve the misrepresentation of breast tumors in the mask image use for training the segmentation model as this could affect the outcome of the training process. We obtained an IOU of 99.0%, and dice similarity score of 94.0. For our approach It could be seen clearly here that our proposed model achieved a better result in terms of Intersection over Union and Dice score similarity.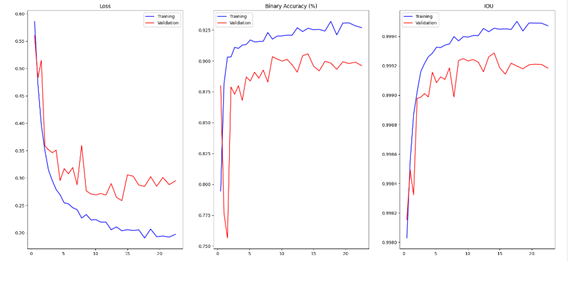
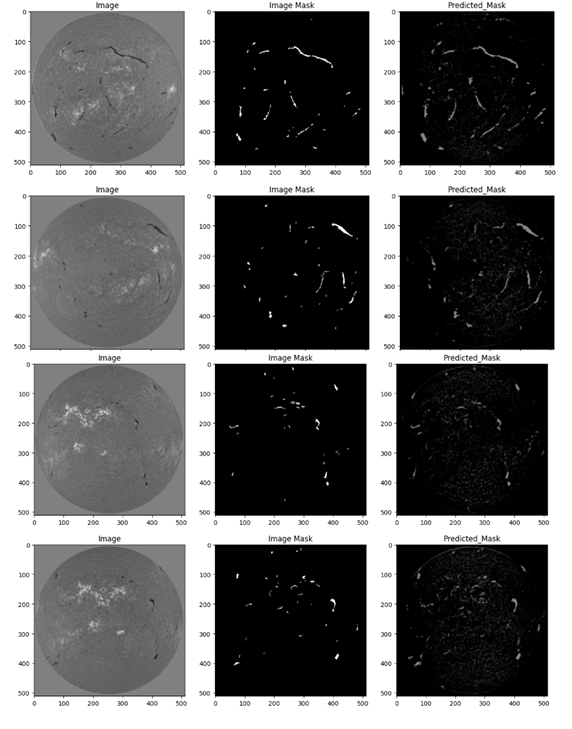