Detection of Tuberculosis Bacilli using Mask R-CNN deep neural network model.
Adebayo Abdulganiyu KEJI || Abdulrahim MOHAMMED
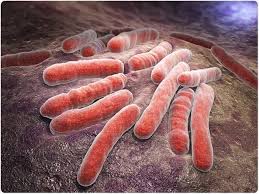
Abstract
Tuberculosis is primarily caused by the bacteria Mycobacterium tuberculosis, which can also affect other organs such as the spine and bones. In severe cases, TB can also cause damage to the kidney and brain. People who have lung tuberculosis can spread the disease through their spit, sneeze, or cough. For someone to get infected, they only need to breathe in a few germs. Antimicrobial resistance is largely caused by tuberculosis (TB), which is also the primary cause of death for individuals living with HIV. Despite being commonplace throughout the world, the majority of TB patients live in low- and middle-income countries. A screening test is necessary to diagnose tuberculosis infection because the infected individuals do not show any symptoms. Pathologists use a range of methods to find tuberculosis. Sputum smear slide requires technicians to evaluate between 20 and 100 fields, which can take anywhere from 40 minutes to three hours depending on the patient's infection level. According to Veropoulos et al., automated methods may help to increase the diagnostic sensitivity rate as machines can screen a large number of fields in a short amount of time and can detect the TB bacillus more accurately in the early stage itself. Manual TB detection and counting methods may miss 33-50% of active cases. Within the landscape of Convolutional Neural Networks (CNNs), the utilization of advanced models such as Mask R-CNN signifies a remarkable stride in image segmentation, showcasing CNN's profound ability to not only detect but accurately delineate and identify the intricate features of TB bacilli within medical imaging In this study, we implemented Mask RCNN object detection model for tuberculosis bacilli detection and compared our model with SVM, KNN, QDA, LDA, Faster R-CNN, Faster RCNN + CNN. The Mask RCNN model performed best with a precision of 85.70%, recall of 99% and 91% F1 score. The Faster RCNN + CNN also gave a competitive result of 85.1% for precision, 98.40% for recall and F1 score, the Faster RCNN also gave 82.60% for precision, 98.30% for recall and F1 score of 98.70%.
Methodology
TThe dataset, the procedure for the experiment, and the assessment standards are all introduced here, along with the methodology that was used to conduct the experiment for the study.
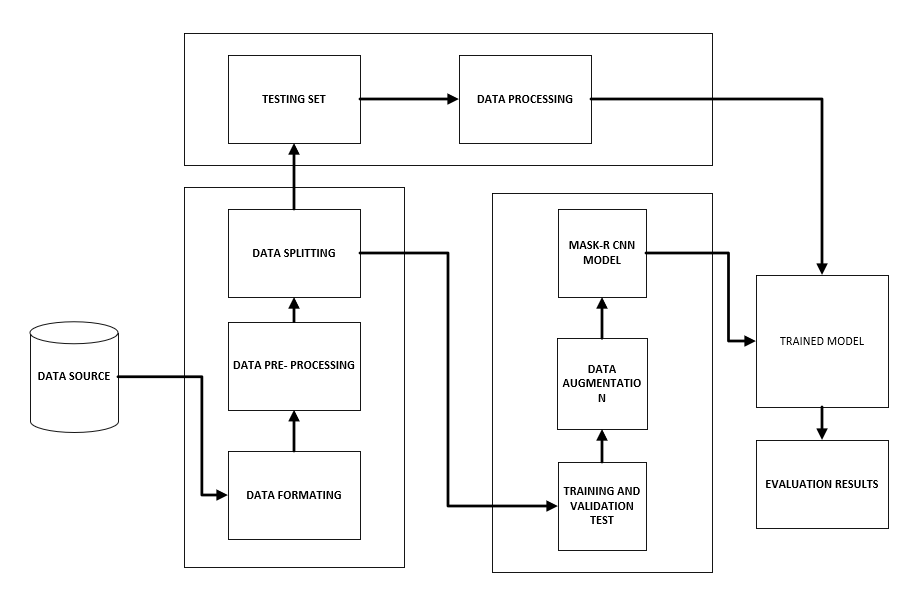
Conclusion
In this study, we implemented Mask RCNN object detection model for tuberculosis bacilli detection and compared our model with SVM, KNN, QDA, LDA, Faster R-CNN, Faster RCNN + CNN. The Mask RCNN model performed best with a precision of 85.70%, recall of 99% and 91% F1 score. The Faster RCNN + CNN also gave a competitive result of 85.1% for precision, 98.40% for recall and F1 score, the Faster RCNN also gave 82.60% for precision, 98.30% for recall and F1 score of 98.70%.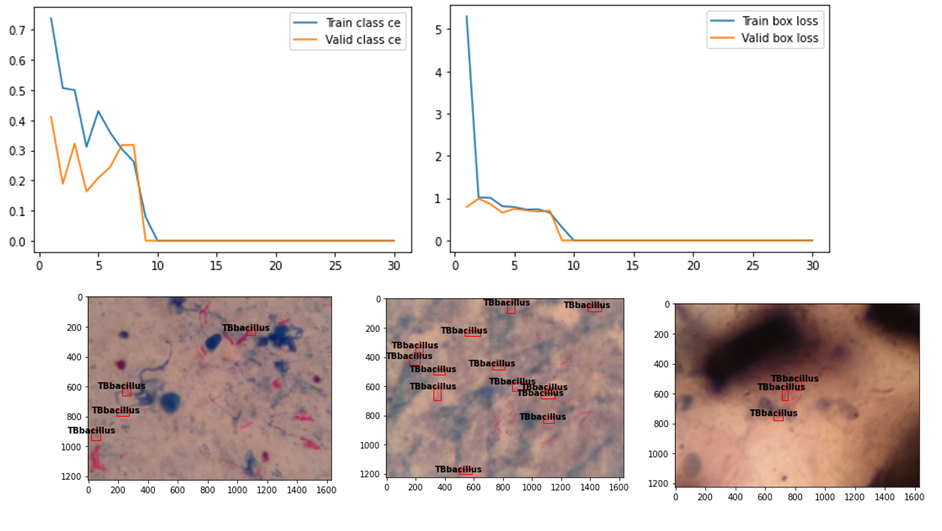