Detection of brain tumors from a computed tomographic - magnetic resonance imagery using segmentation deep learning technique.
Adebayo Abdulganiyu KEJI || Nnamdi Bobby Nwaekwu
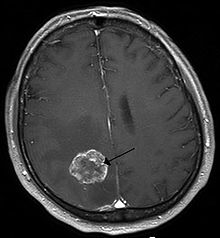
Abstract
The leading cause of cancer death in children and adults under 40 is brain tumors [1]. A primary brain tumor affects more than 12,000 individuals a year, including 500 children and teenagers; that's 33 people every day. Brain imaging technology continues to be at the forefront of developments in both comprehension of the brain and the capacity to identify and treat brain diseases and illnesses [2]. The automated triage of imaging scans using computer algorithms has the potential to identify brain tumors earlier with high degree of precision and accuracy thereby improving therapeutic outcomes. In this study, we propose the use of hybrid segmentation model known as SegUnet which has the capacity to address the issue of small size tumors getting lost during down sampling to train an MRI-CT cross modality dataset. In addition to this, we research on the effectiveness of filter size in the segmentation of medical images. However, we implemented three different SegUnet models with modification on filter size. We employed size 2, 3 and 4 to test the efficiency of filter on medical image segmentation. The report of the result shows that the model with filter size of 2 and size of 3 almost have the same evaluation results in terms of mean pixel accuracy, global accuracy, IOU and frequency weighted IU while model with filter size of 4 is relatively lesser in metrics compared to the others. Also, looking closely at the training and loss plot of the respective filters it could be noted that the model of filter size 3 loss plot was stable and decreases steadily while the others are in zig-zag pattern or better put as unstable. More so, the training plot of filter size 3 was reasonably stable compared to the other two models. Thus, we conclude that our model with filter size of 3 performed better compared to the other two models and [3] model in terms of generalization as we used two different image modalities for the training of our model.
Methodology
This introduces the methodology that was utilized to carry out the study for this thesis, along with the dataset, the experiment's workflow, and the evaluation criteria.
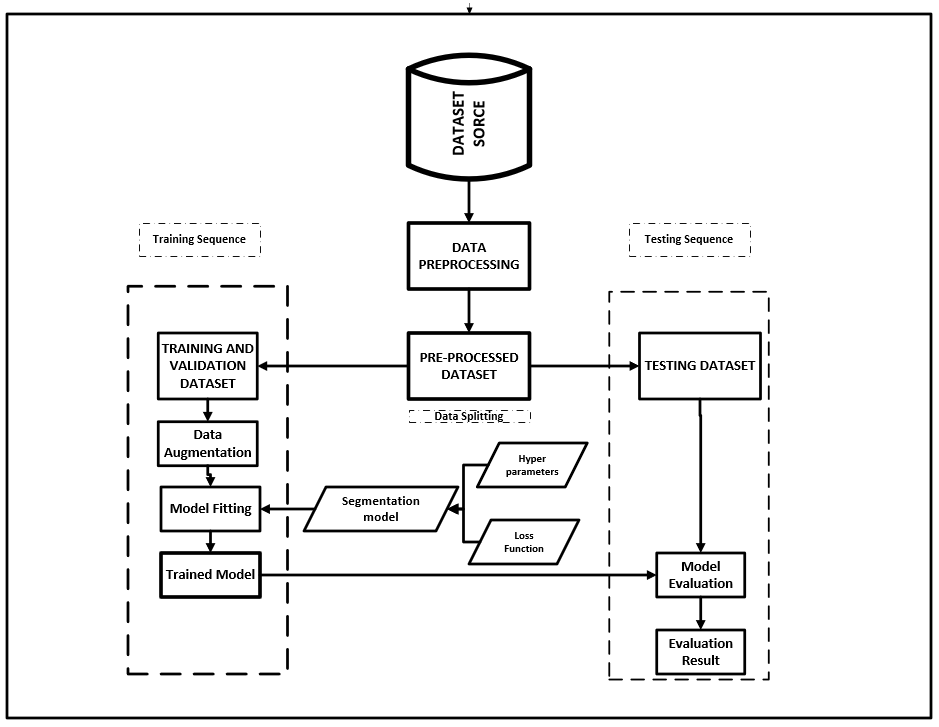
Conclusion
In this study, we proposed the use of a hybrid segmentation model known as SegUnet. The reason why we propose the use of this model in our study was because of the capability of the model to address the issue of small size tumors getting lost during down sampling, which results in inappropriate segmentation as seen in [3]. While studies like [3] have employed the use of this model in developing brain tumor segmentation using MRI images no study have employed the use of two or more modalities in training the model. Thus, we employed the use of MRI-CT image modalities from the just released dataset seen in [20] to train our SegUnet hybrid segmentation model. In addition to this we research on the effectiveness of filter size in the segmentation of medical images. However, we implemented three different SegUnet model with modification on filter size. We employed size 2, 3 and 4 to test the efficiency of filter on medical image segmentation. We obtained 99.09% for mean pixel accuracy, a global accuracy of 95.22%, IOU of an 83.94% and 98.47% of frequency weighted UI for model with filter size of 2 and obtained 99.14% for mean pixel accuracy, a global accuracy of 94.62%, IOU of an 83.47% and 98.51% of frequency weighted UI for model with filter size of 3 while for model with filter size of 4 we obtained 98.74% for mean pixel accuracy, a global accuracy of 94.40%, IOU of an 80.05% and 97.90% of frequency weighted UI. It could be deduced from the report of the result that the model with filter size of 2 and size of 3 almost have the same evaluation results in terms of mean pixel accuracy, global accuracy, IOU and frequency weighted IU while model with filter size of 4 is relatively lesser in metrics compared to the others. Also, looking closely at the training and loss plot of the respective filters it could be noted that the model of filter size 3 loss plot was stable and decreases steadily while the others are in zig-zag pattern or better put as unstable. More so, the training plot of filter size 3 was reasonably stable compared to the other two models. Thus, we could conclude that our model with filter size of 3 performed better compared to the other two models and [3] model in terms of generalization as we used two different image modalities for the training of our model.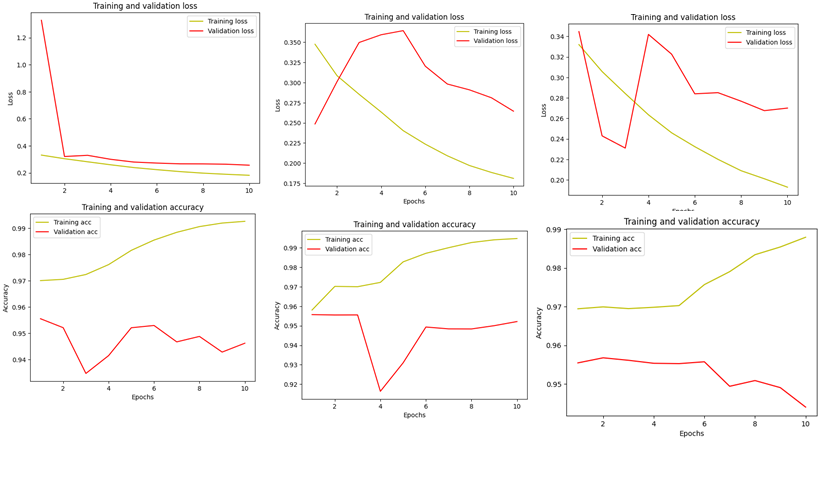
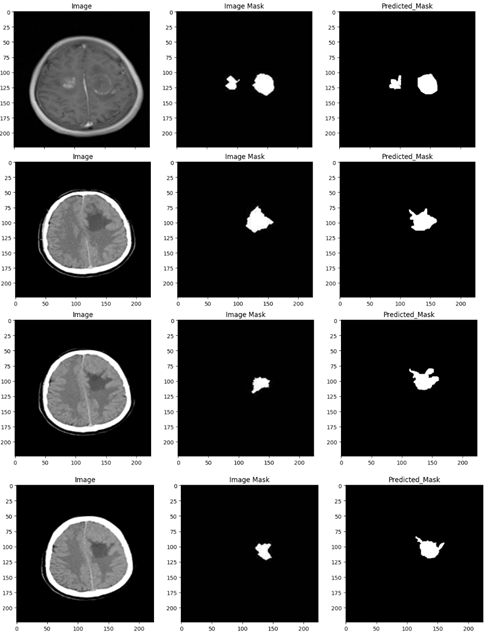