Application of deep neural network for object detection in augmented reality.
Adebayo Abdulganiyu KEJI || Olufemi JOHNSON
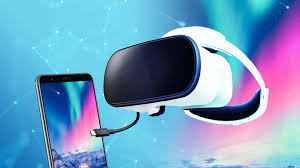
Abstract
Augmented reality (AR) is a cutting-edge technology that has advanced dramatically in recent years, opening up a multitude of opportunities and applications that have revolutionized several industries. The majority of marker-based augmented reality applications available today rely on local feature tracking and detection methods which gives room for improvement in the performance of AR in terms of speed and mean precision. The application of DNN for augmented reality object detection opens up a world of possibilities. by enabling AR systems to interact and comprehend the real world at a high degree of accuracy and object detection rate. In this study we developed a deep neural network (YOLOv3) model for object detection in augmented reality. The model achieved a mean Average Precision (mAP) of 77.8% and average execution time of about 0.29756 and dice coefficient score of around 0.8814 while in the work of Oufkir et.al [30] a mean average precision of 72.7% was achieved and average execution time of 0.27100 and 0.8614 average dice coefficient similarity score. However, our model performs better in terms of mAP and DSC score while the execution time of their model is quite better than ours but this can be attributed to the android software used for performing the detection.
Methodology
This introduces the methodology that was utilized to carry out the study for this thesis, along with the dataset, the experiment's workflow, and the evaluation criteria.
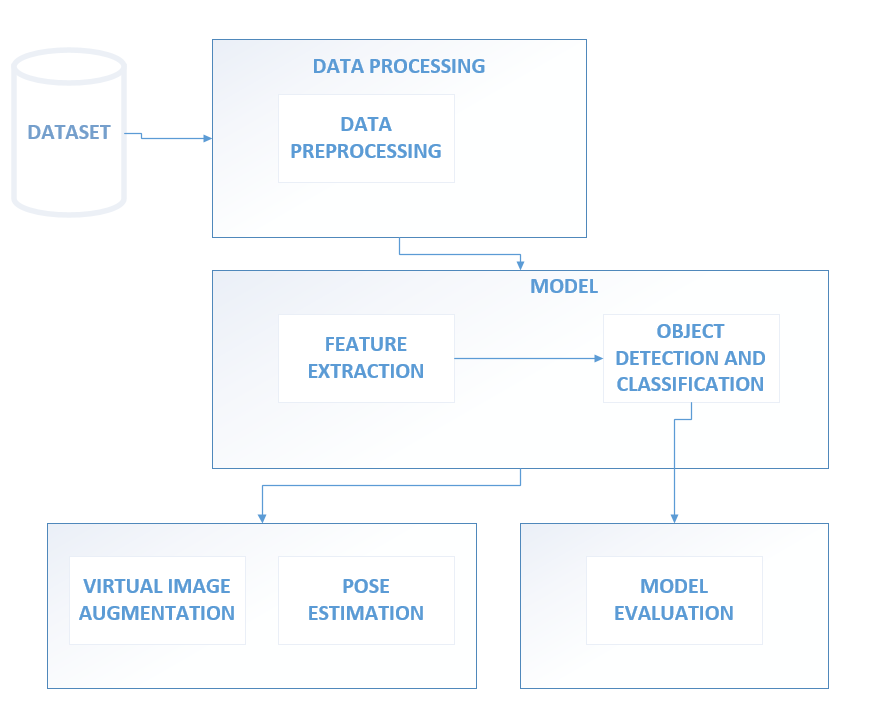
Conclusion
In this study we developed a deep neural network object detection model for use in augmented reality. The model achieved a mean average precision (mAP) of 77.8% and average execution time of about 0.29756 and dice coefficient score of around 0.8814 while in the work of Oufkir et.al [30] a mean average precision of 72.7% was achieved and average execution time of 0.27100 and 0.8614 average dice coefficient similarity score. However, our model perform better in terms of mAP and DSC score while the execution time of their model is quite better than ours but this can be attributed to the android software used for performing the detection.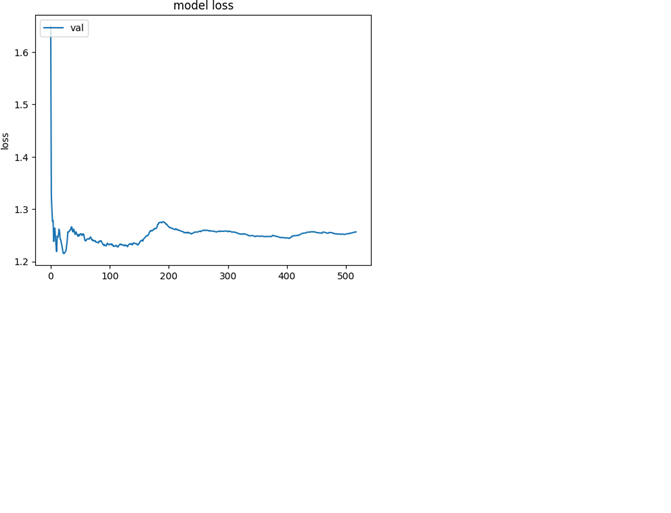
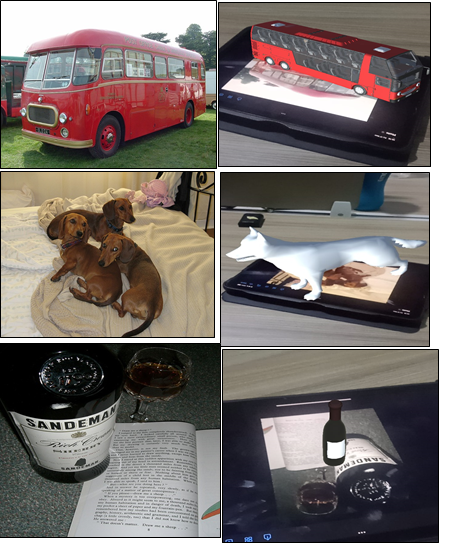